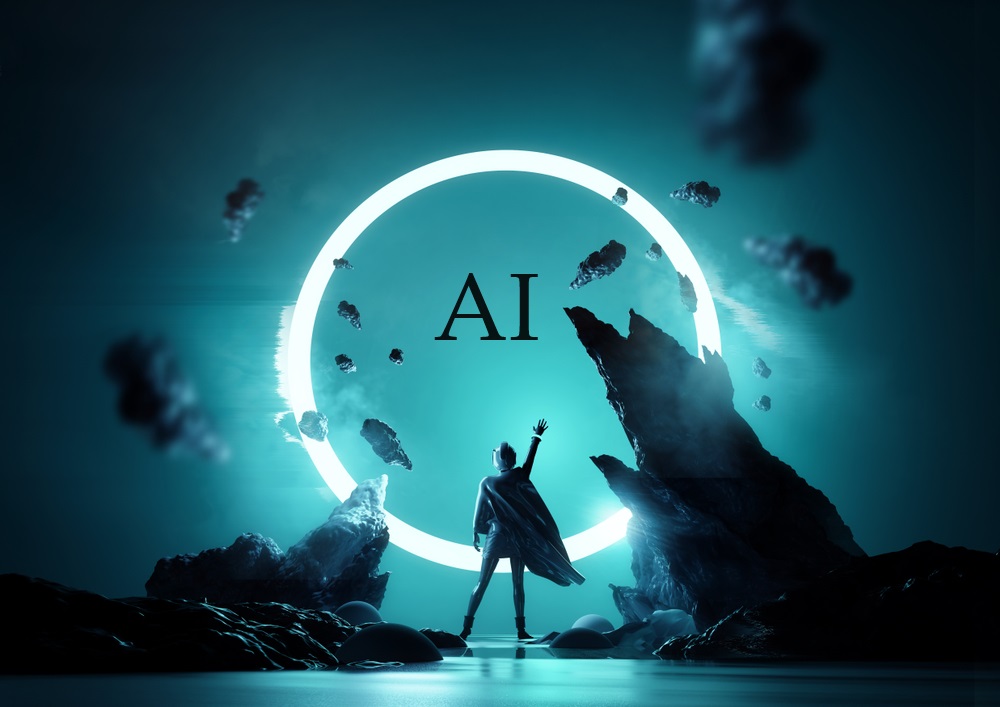
(solarseven/Shutterstock)
As the worldwide generative AI rollout unfolds, corporations are grappling with a bunch of moral and governance considerations: Ought to my workers worry for his or her jobs? How do I make sure the AI fashions are adequately and transparently educated? What do I do about hallucinations and toxicity? Whereas it’s not a silver bullet, holding people within the AI loop is an effective solution to deal with an honest cross-section of AI worries.
It’s outstanding how a lot progress has been made in generative AI since OpenAI shocked the world with the launch of ChatGPT only a year-and-a-half in the past. Whereas different AI tendencies have come and gone, massive language fashions (LLMs) have caught the eye of technologists, enterprise leaders, and shoppers alike.
Firms collectively are investing trillions of {dollars} to get a leg up in GenAI, which is forecasted to create trillions in new worth in only a matter of years. And whereas there was a little bit of a pullback recently, many are banking that we’ll see large returns on funding (ROI), similar to the brand new Google Cloud examine that discovered 86% of GenAI adopters are seeing a development of 6% or extra in annual firm income.
So What’s the Maintain Up?
We’re at an attention-grabbing level within the GenAI revolution. The know-how has proved that it’s largely prepared, and early adopters are reporting some success. What’s holding up the massive GenAI success celebrations, it might appear, are a number of the knottier questions round issues like ethics, governance, safety, privateness, and rules.
In different phrases, we will implement GenAI. However the large query is ought to we? If the reply to that questions is “sure,” the following one is: So how can we implement it whereas adhering to requirements round ethics, governance, safety, and privateness, to say nothing of recent rules, just like the EU AI Act?
For some perception into the matter, Datanami spoke to Cousineau, the vp of knowledge mannequin and governance at Thomson Reuters. The Toronto, Ontario-based firm has been within the info enterprise for practically a century, and final 12 months, its 25,000-plus employs helped the corporate usher in about $6.8 billion in income throughout 4 divisions, together with authorized, tax and accounting, authorities, and the Reuters Information Company.
As the pinnacle of Thomson Reuters’ accountable AI follow, Cousineau has substantial affect on how the publicly traded firm implements AI. When she first took the place in 2021, her first objective was to implement a company-wide program to centralize and standardize the way it builds accountable and moral AI.
As Cousineau explains, she began out by main her staff to ascertain a set of rules for AI and knowledge. As soon as these rules had been in place, they then devised a collection of insurance policies and procedures to information how these rules can be carried out in follow, together with with each new AI and knowledge methods in addition to legacy methods.
When ChatGPT landed on the world in late November 2022, Thomson Reuters was prepared.
“We did have a very good chunk of time [to build this] earlier than generative AI took off,” she says. “But it surely allowed us to have the ability to react faster as a result of we had the foundational work performed and this system operate, so we didn’t need to begin to attempt to create that. We truly simply needed to constantly refine these management factors and implementations, and we nonetheless do on account of generative AI.”
Constructing Accountable AI
Thomson Reuters isn’t any stranger to AI, and the corporate has been working with some type of AI, machine studying, and pure language processing (NLP) for many years earlier than Cousineau arrived. The corporate had “notoriously…nice practices” in place” round AI, she says. What it was lacking, nonetheless, was the centralization and standardization wanted to get to the following stage.
Information impression assessments (DIAs) are a important means the corporate stays on prime of potential AI threat. Working along side Thomson Reuters legal professionals, Cousineau’s staff does an exhaustive evaluation of the dangers of a proposed AI use case, from the kind of knowledge that’s concerned and the proposed algorithm, to the area and naturally the meant use.
“The panorama total is completely different relying on the jurisdiction, from a legislative standpoint. That’s why we work so carefully with the overall counsel’s workplace as nicely,” Cousineau says. “However to construct the sensible implementation of moral idea into AI methods, our candy spot is working with groups to place the precise controls in place, prematurely of what regulation is anticipating us to do.”
Cousineau’s staff construct a handful of recent inner instruments to assist the information and AI groups keep on the straight and slender. As an example, it developed a centralized mannequin repository, the place a report of the entire firm’s AI fashions was saved. Along with boosting the productiveness of Thomson Reuters’ 4,300 knowledge scientists and AI engineers, who’ve a better solution to uncover and re-use fashions, it additionally allowed Cousineau’s staff to layer governance on prime. “It’s a twin profit that it served,” she says.
One other necessary instrument is the Accountable AI Hub, the place the particular dangers related to an AI use case are laid out and the completely different groups can work collectively to mitigate the challenges. These mitigations may very well be a chunk of code, a verify, or perhaps a new course of, relying on the character of the danger (similar to privateness, copyright violation, and so forth.).
However for different sorts of AI functions, the most effective methods of making certain accountable AI is by holding people within the loop.
People within the Loop
Thomson Reuters has numerous nice processes for mitigating AI threat, even in area of interest environments, Cousineau says. However relating to holding people within the loop, the corporate advocates taking a multi-pronged method that ensures human participation on the design, growth, and deployment levels, she says.
“One of many management factors we’ve got in mannequin documentation is an precise human oversight description that the builders and product homeowners would put collectively,” she says. “As soon as it strikes to deployment, there are [several] methods you’ll be able to take a look at it.”
As an example, people are within the loop relating to guiding how purchasers and clients use Thomson Reuters merchandise. There are additionally groups on the firm devoted to offering human-in-the-loop coaching, she says. It additionally locations disclaimers in some AI merchandise reminding customers that the system is simply for use for analysis functions.
“Human within the loop is a really heavy idea that we combine all through,” Cousineau says. “And even as soon as it’s out of deployment, we use [humans in the loop] to measure.”
People play a important function in monitoring AI fashions and AI functions at Thomson Reuters, together with issues like monitoring mannequin drift, monitoring the general efficiency of fashions, together with precision, recall, and confidence scores. Material excerpts and legal professionals additionally evaluate the output of its AI methods, she says.
“Having human reviewers is part of that system,” she says. “That’s the piece the place a human within the loop side will constantly play a vital function for organizations, as a result of you may get that consumer suggestions with a view to make sure that the mannequin’s nonetheless performing the way in which wherein you meant it to be. So people are actively nonetheless within the loop there.”
The Engagement Issue
Having people within the loop doesn’t simply make the AI methods higher, whether or not the measure is bigger accuracy, fewer hallucinations, higher recall, fewer privateness violations. It does all these issues, however there’s another necessary issue that enterprise homeowners will need to remember: It reminds staff that they’re important to the success of the corporate, and that AI gained’t change them.
“That’s the half that’s attention-grabbing about human within the loop, the invested curiosity to have that human energetic engagement and in the end nonetheless have the management and possession of that system. [That’s] the place nearly all of the consolation is.”
Cousineau remembers attending a current roundtable on AI hosted by Snowflake and Cohere with executives from Thomson Reuters and different corporations, the place this query got here up. “No matter the sector…they’re all comfy with understanding that they’ve a human within the loop,” she says. “They don’t want a human out of the loop, and I don’t see why they might wish to, both.”
As corporations chart their AI futures, enterprise leaders might want to strike a steadiness between humanness and AI. That’s one thing they’ve needed to do with each technological enchancment over the previous two thousand years.
“What a human within the loop will present is the data of what the system can and may’t do, after which you need to optimize this to your benefit,” Cousineau says. “There are limitations in any know-how. There are limitations in doing issues fully manually, completely. There’s not sufficient time within the day. So it’s discovering that steadiness after which having the ability to have a human within the loop method, that may be one thing that everybody is prepared for.”
Associated Gadgets:
5 Questions because the EU AI Act Goes Into Impact
What’s Holding Up the ROI for GenAI?
AI Ethics Nonetheless In Its Infancy