Introduction
You understand how we’re all the time listening to about “various” datasets in machine studying? Effectively, it turns on the market’s been an issue with that. However don’t fear – a superb staff of researchers has simply dropped a game-changing paper that’s received the entire ML neighborhood buzzing. Within the paper that lately gained the ICML 2024 Finest Paper Award, researchers Dora Zhao, Jerone T. A. Andrews, Orestis Papakyriakopoulos, and Alice Xiang sort out a vital situation in machine studying (ML) – the usually obscure and unsubstantiated claims of “variety” in datasets. Their work, titled “Measure Dataset Variety, Don’t Simply Declare It,” proposes a structured strategy to conceptualizing, operationalizing, and evaluating variety in ML datasets utilizing rules from measurement principle.
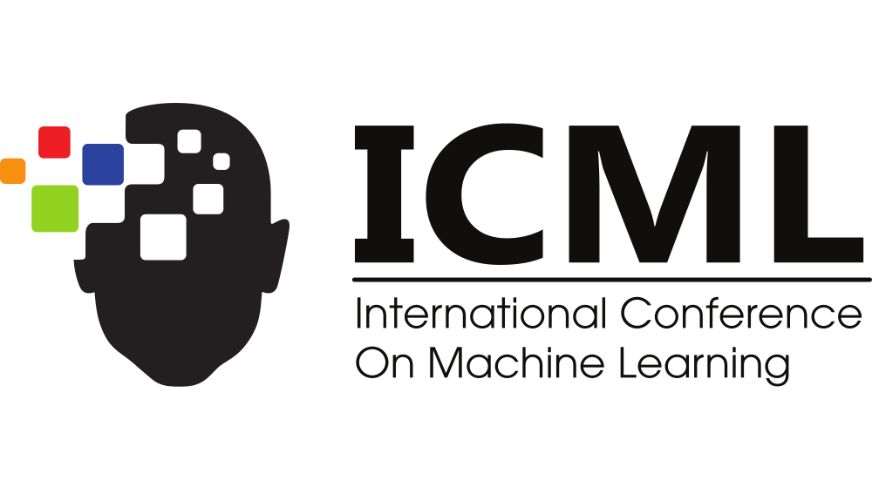
Now, I do know what you’re considering. “One other paper about dataset variety? Haven’t we heard this earlier than?” However belief me, this one’s completely different. These researchers have taken a tough have a look at how we use phrases like “variety,” “high quality,” and “bias” with out actually backing them up. We’ve been taking part in quick and unfastened with these ideas, they usually’re calling us out on it.
However right here’s one of the best half—they’re not simply stating the issue. They’ve developed a stable framework to assist us measure and validate variety claims. They’re handing us a toolbox to repair this messy state of affairs.
So, buckle up as a result of I’m about to take you on a deep dive into this groundbreaking analysis. We are going to discover how we will transfer past claiming variety to measuring it. Belief me, by the tip of this, you’ll by no means have a look at an ML dataset the identical approach once more!
The Drawback with Variety Claims
The authors spotlight a pervasive situation within the Machine studying neighborhood: dataset curators incessantly make use of phrases like “variety,” “bias,” and “high quality” with out clear definitions or validation strategies. This lack of precision hampers reproducibility and perpetuates the misunderstanding that datasets are impartial entities reasonably than value-laden artifacts formed by their creators’ views and societal contexts.
A Framework for Measuring Variety
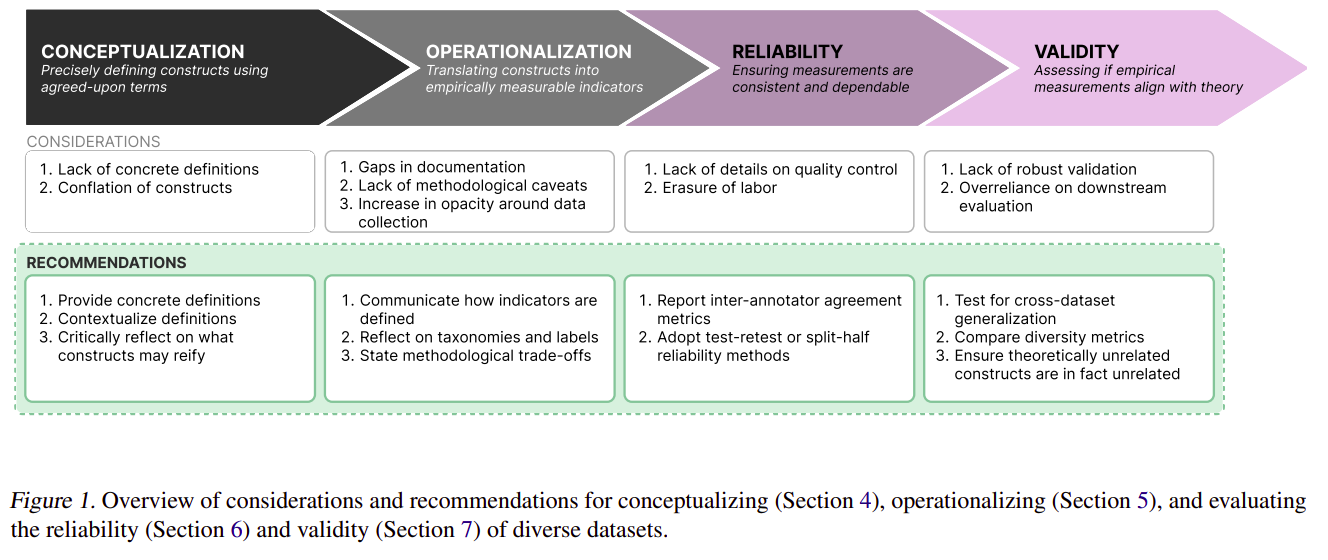
Drawing from social sciences, notably measurement principle, the researchers current a framework for reworking summary notions of variety into measurable constructs. This strategy includes three key steps:
- Conceptualization: Clearly defining what “variety” means within the context of a particular dataset.
- Operationalization: Growing concrete strategies to measure the outlined features of variety.
- Analysis: Assessing the reliability and validity of the range measurements.
In abstract, this place paper advocates for clearer definitions and stronger validation strategies in creating various datasets, proposing measurement principle as a scaffolding framework for this course of.
Key Findings and Suggestions
Via an evaluation of 135 picture and textual content datasets, the authors uncovered a number of essential insights:
- Lack of Clear Definitions: Solely 52.9% of datasets explicitly justified the necessity for various knowledge. The paper emphasizes the significance of offering concrete, contextualized definitions of variety.
- Documentation Gaps: Many papers introducing datasets fail to supply detailed details about assortment methods or methodological selections. The authors advocate for elevated transparency in dataset documentation.
- Reliability Considerations: Solely 56.3% of datasets coated high quality management processes. The paper recommends utilizing inter-annotator settlement and test-retest reliability to evaluate dataset consistency.
- Validity Challenges: Variety claims usually lack sturdy validation. The authors counsel utilizing strategies from assemble validity, reminiscent of convergent and discriminant validity, to guage whether or not datasets actually seize the supposed variety of constructs.
Sensible Utility: The Phase Something Dataset
As an instance their framework, the paper features a case examine of the Phase Something dataset (SA-1B). Whereas praising sure features of SA-1B’s strategy to variety, the authors additionally determine areas for enchancment, reminiscent of enhancing transparency across the knowledge assortment course of and offering stronger validation for geographic variety claims.
Broader Implications
This analysis has vital implications for the ML neighborhood:
- Difficult “Scale Considering”: The paper argues in opposition to the notion that variety routinely emerges with bigger datasets, emphasizing the necessity for intentional curation.
- Documentation Burden: Whereas advocating for elevated transparency, the authors acknowledge the substantial effort required and name for systemic modifications in how knowledge work is valued in ML analysis.
- Temporal Concerns: The paper highlights the necessity to account for a way variety constructs might change over time, affecting dataset relevance and interpretation.
You possibly can learn the paper right here: Place: Measure DatasetOkay Variety, Don’t Simply Declare It
Conclusion
This ICML 2024 Finest Paper provides a path towards extra rigorous, clear, and reproducible analysis by making use of measurement principle rules to ML dataset creation. As the sector grapples with problems with bias and illustration, the framework introduced right here offers beneficial instruments for guaranteeing that claims of variety in ML datasets will not be simply rhetoric however measurable and significant contributions to growing honest and sturdy AI techniques.
This groundbreaking work serves as a name to motion for the ML neighborhood to raise the requirements of dataset curation and documentation, in the end resulting in extra dependable and equitable machine studying fashions.
I’ve received to confess, once I first noticed the authors’ suggestions for documenting and validating datasets, part of me thought, “Ugh, that seems like lots of work.” And yeah, it’s. However what? It’s work that must be carried out. We will’t preserve constructing AI techniques on shaky foundations and simply hope for one of the best. However right here’s what received me fired up: this paper isn’t nearly enhancing our datasets. It’s about making our total discipline extra rigorous, clear, and reliable. In a world the place AI is turning into more and more influential, that’s big.
So, what do you suppose? Are you able to roll up your sleeves and begin measuring dataset variety? Let’s chat within the feedback – I’d love to listen to your ideas on this game-changing analysis!
You possibly can learn different ICML 2024 Finest Paper‘s right here: ICML 2024 High Papers: What’s New in Machine Studying.
Incessantly Requested Questions
Ans. Measuring dataset variety is essential as a result of it ensures that the datasets used to coach machine studying fashions characterize numerous demographics and situations. This helps cut back biases, enhance fashions’ generalizability, and promote equity and fairness in AI techniques.
Ans. Numerous datasets can enhance the efficiency of ML fashions by exposing them to a variety of situations and lowering overfitting to any explicit group or situation. This results in extra sturdy and correct fashions that carry out properly throughout completely different populations and circumstances.
Ans. Widespread challenges embrace defining what constitutes variety, operationalizing these definitions into measurable constructs, and validating the range claims. Moreover, guaranteeing transparency and reproducibility in documenting the range of datasets might be labor-intensive and sophisticated.
Ans. Sensible steps embrace:
a. Clearly defining variety objectives and standards particular to the mission.
b. Amassing knowledge from numerous sources to cowl completely different demographics and situations.
c. Utilizing standardized strategies to measure and doc variety in datasets.
d. Repeatedly consider and replace datasets to keep up variety over time.
e.Implementing sturdy validation strategies to make sure the datasets genuinely mirror the supposed variety.